|
Multimodal
Neuroimaging
Multimodal brain imaging is a promising and
recent approach to study the function and
structure of the brain by collecting multiple
types of data from the same individual using
various imaging techniques (fMRI, fNIRS, MRI,
DTI, EEG, etc.). This allows the examination
of complex brain functions and diseases from
different imaging techniques, which can yield
important insights that are only partially
detected by each modality. At the same time,
however, the advent of multimodal neuroimaging
has brought the urgent need to develop new
data integration methods that can capitalize
on the strength of each modality and make full
usage of the data dimensionality and
informational complexity
In one of our projects, we utilized single and
multimodal brain imaging data including MRI,
DTI, and rs‐fMRI from a large data set with
839 subjects to predict brain age. We
evaluated the prediction performance of 36
different combinations of imaging features and
ML models including deep learning. We showed
that multimodal brain imaging features derived
from MRI, DTI, and rs‐fMRI yielded higher
brain age prediction accuracy than
single‐modal features. See here
for more details.
|
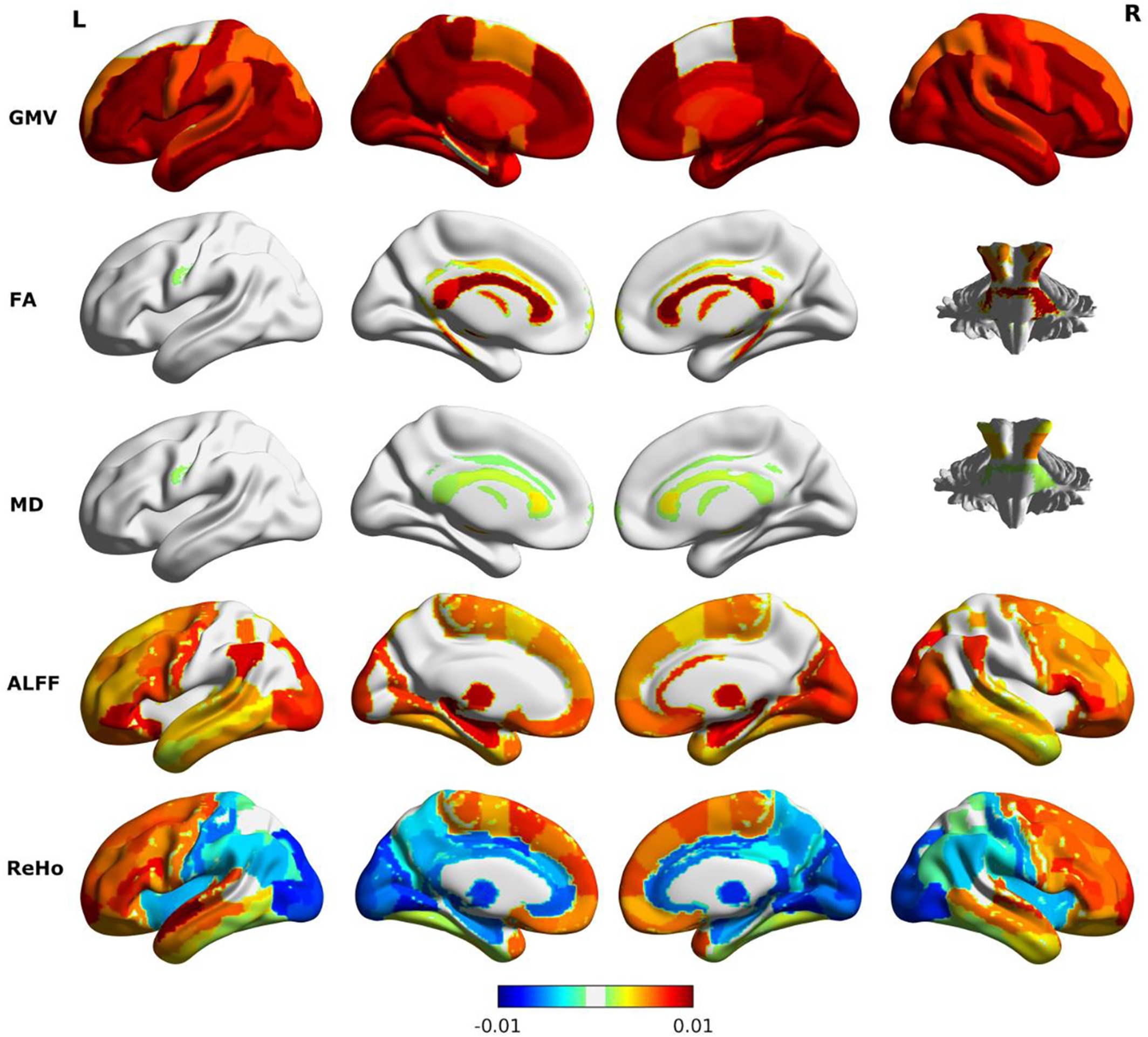
|
Identifying
antemortem biomarkers for progression from
mild cognitive impairment (MCI) to dementia of
Alzheimer‘s type is crucial to detect areas
particularly sensitive to neurodegeneration
caused by Alzheimer’s disease (AD), in
addition to potentially providing a strong
diagnostic tool at early disease stages. In
several of our projects, we examined the
transition probability between MCI and
dementia using imaging features from multiple
modalities (e.g., MRI, FDG-PET). To deal with
a large number of imaging features, we
incorporate penalization in multi-state Markov
transition models to select predictive imaging
signatures of AD. See here
for details.
In another project, we used multimodal
magnetic resonance imaging (MRI) images (i.e.,
T1-w, T2-w, PD-w, and FLAIR) to detect
multiple sclerosis (MS) lesions. Accurate
identification of MS lesions is essential for
MS diagnosis, assessment of disease
progression, and evaluation of treatment
efficacy. However, it is a challenging task
due to the variability in lesion location,
size and shape in addition to anatomical
variability between subjects. In this work, we
developed an integrative classification model
that incorporates information from different
resources to achieve robust and reliable
segmentation results. Click here
for details.
|
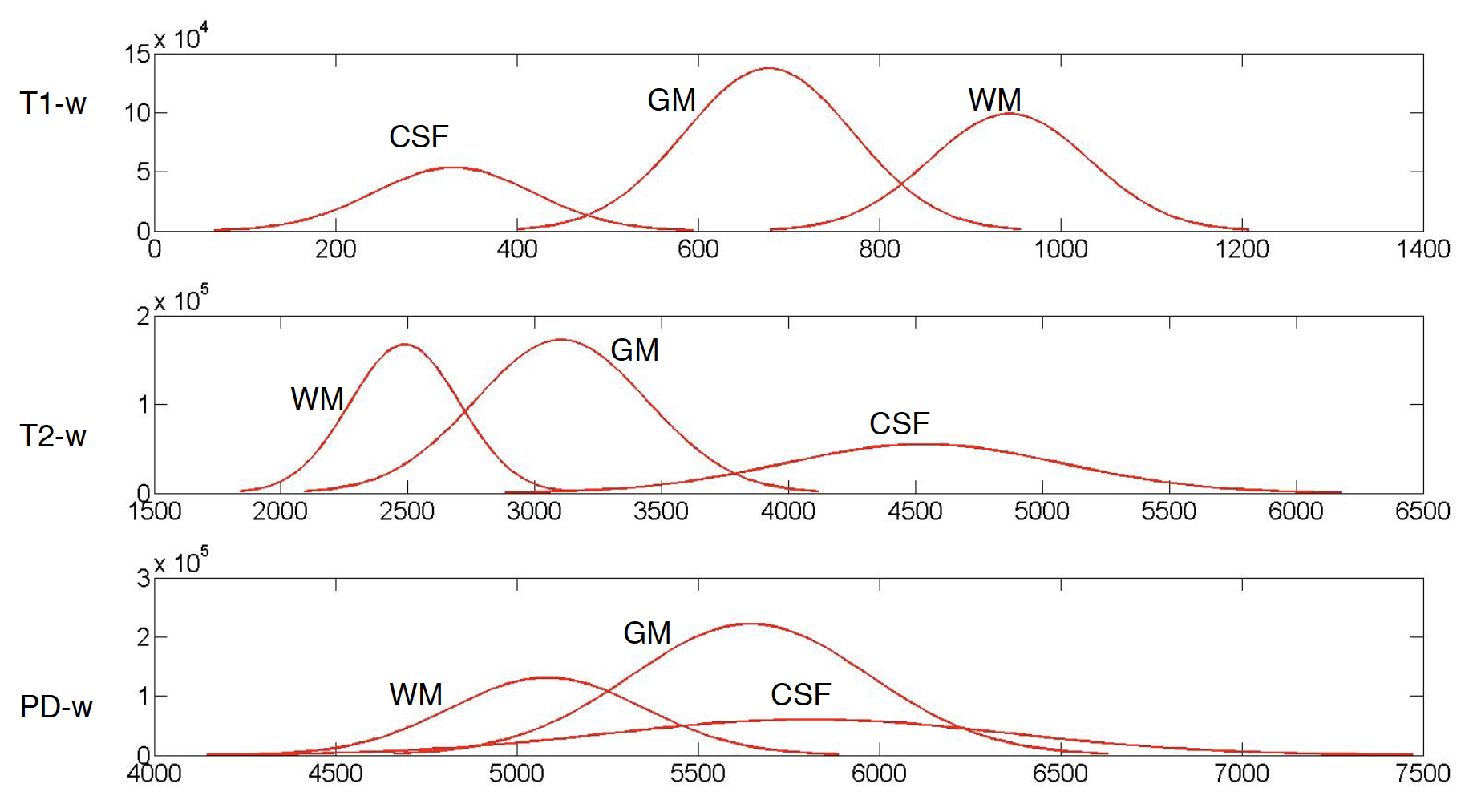
|
Brain
Age Prediction
Brain age prediction based on imaging data and
machine learning (ML) methods has great
potential to provide insights into the
development of cognition and mental disorders.
Using machine learning (ML) methods, an age
prediction model is first built with brain
imaging features from a training data set and
then applied to estimate the age of new
individuals in an independent test set. The
predicted age based on brain imaging data is
often referred to as brain age. The difference
between predicted age and chronological age is
considered as the brain age gap, which is
helpful for quantifying delayed or advanced
development for youth as well as accelerated
or slowed aging for elderly people.
In several projects, we investigated brain age
prediction with different ML models and brain
features from multiple imaging modalities. As
the true brain age is unknown and may deviate
from chronological age, we further examine
whether various levels of behavioral
performance across subjects predict their
brain age estimated from neuroimaging data.
This is an important step to quantify the
practical implication of brain age prediction.
Our findings are helpful to advance the
practice of optimizing different analytic
methodologies in brain age prediction. In
addition, we applied the proposed brain age
prediction model to a group of adolescents
with anxiety disorders and tested whether
patients with anxiety disorders showed altered
brain development compared to healthy
controls. See here
for details. We also examined systematic bias
in brain age estimation with application to
post-traumatic stress disorders. Click here
for details.
|
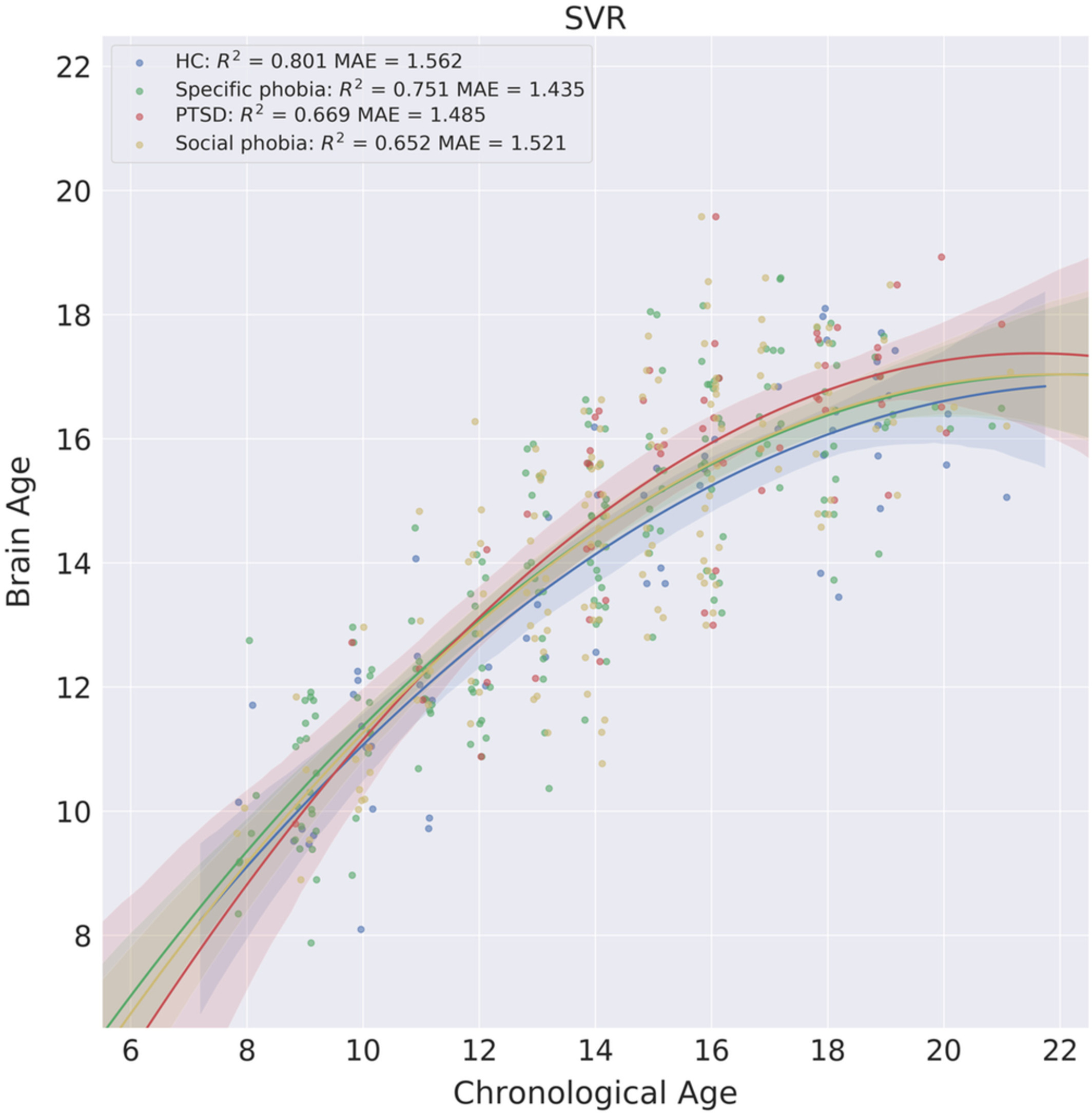
|
Understanding brain age is important for
understanding and diagnosing age-related
neurological disorders so they can be detected
and treated early. It is also important
for understanding how neurological disorders,
injuries, and environmental insults may
prematurely age a brain and how particular
lifestyles may preserve or enhance it
(Cole et al., 2019). We developed a new
EEG-based machine-learning technique for
accurately assessing whether an individual’s
brain is aging more quickly or more slowly than
is typical for healthy individuals. Click here
for details.
|
Neuroimaging
Statistics
Recent advances in human neuroimaging have
shown that it is possible to accurately decode
how the brain perceives information based only
on non-invasive function magnetic resonance
imaging (fMRI) measurements of brain activity.
Two common statistical approaches include
univariate analysis and multivariate pattern
analysis (MVPA).
In one of our projects, we investigated the
representation and processing of auditory
categories within the human temporal cortex
using high resolution fMRI and MVPA methods.
More importantly, we considered decoding
multiple sound categories simultaneously
through multi-class support vector
machine-recursive feature elimination
(MSVM-RFE) as our MVPA tool. Results show that
the model MSVM-RFE was able to learn the
functional relation between the multiple sound
categories and the corresponding evoked
spatial patterns for both within-subject and
across-subject classifications. See here
for more details.
|
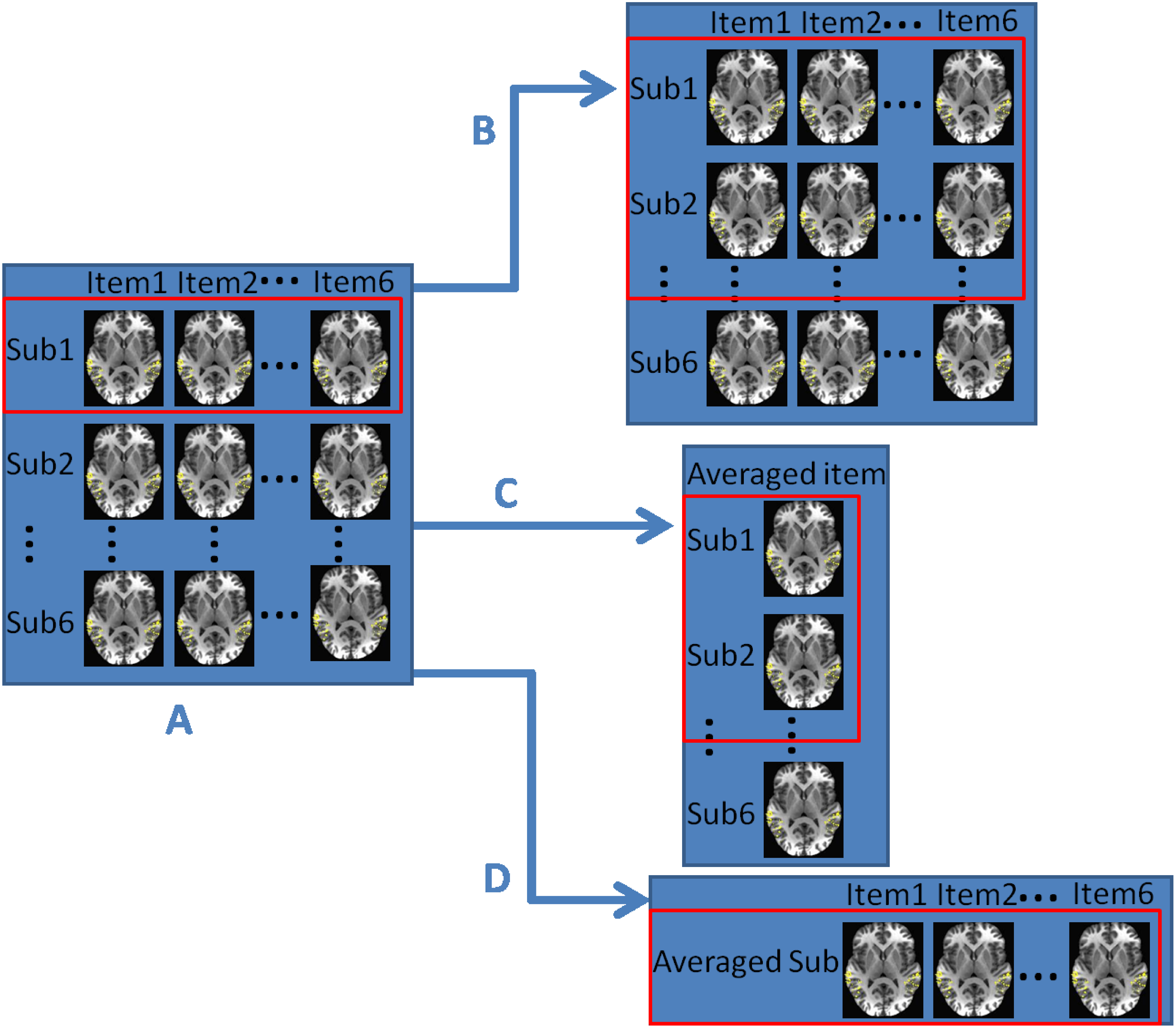
|
Random field theory (RFT) provided a
theoretical foundation for
cluster-extent-based thresholding, the most
widely used method for multiple comparison
correction of statistical maps in neuroimaging
research. However, several studies questioned
the validity of the standard clusterwise
inference in fMRI analyses and observed
inflated false positive rates.
In particular, Eklund et al. used
resting-state fMRI as null data and found
false positive rates of up to 70%, which
immediately led to many discussions. In one of
our projects, we summarized the assumptions in
RFT clusterwise inference and proposed new
parametric ways to approximate the
distribution of the cluster size by properly
combining the limiting distribution of the
cluster size given by Nosko and the expected
value of the cluster size provided by Friston
et al.. We evaluated our proposed method using
four different classic simulation settings in
published papers. Results show that our method
produces a more stringent estimation of
cluster extent size, which leads to a better
control of false positive rates. Click here
for details.
|
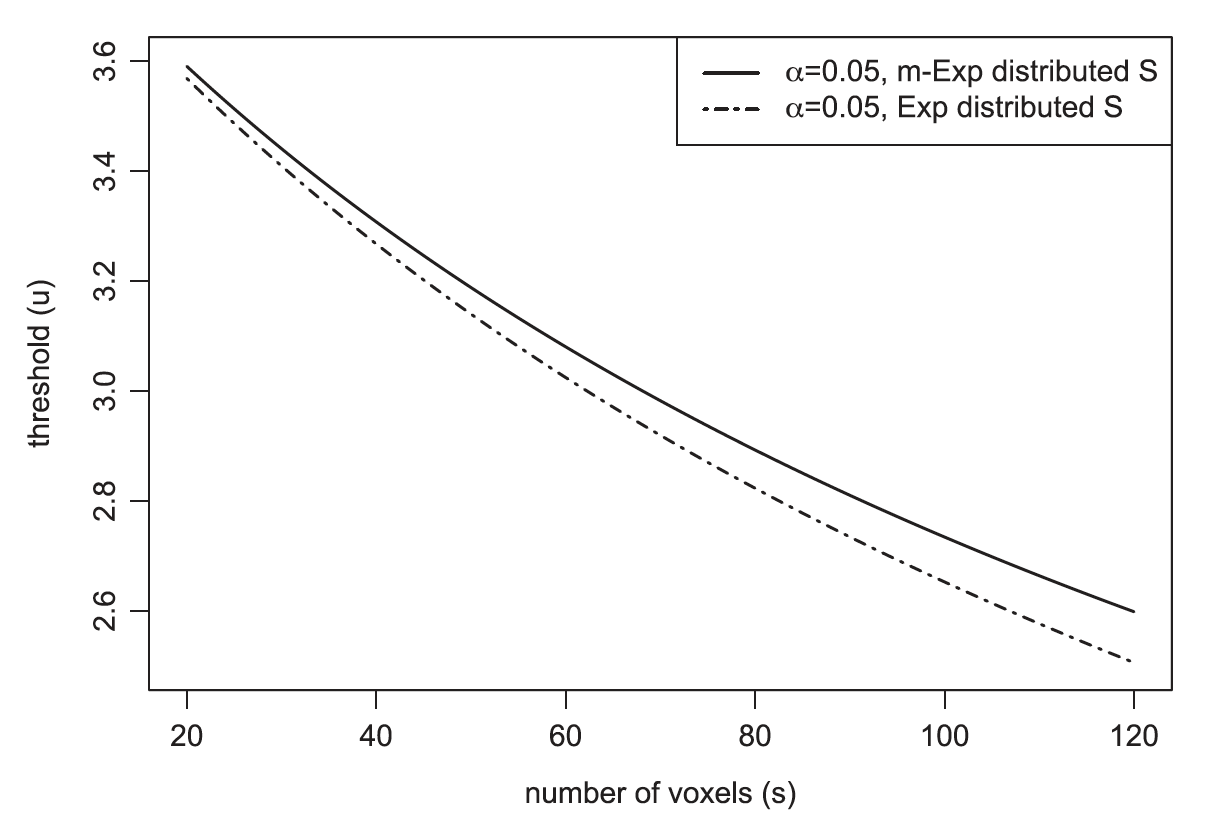
|
Wearable Computing
The integrated measurement of diet, physical
activity, and the built environment is another
important application of big data integration.
Recent advances in wearable computing through
the use of accelerometers, smartphones, and
other devices for tracking individuals and
individual behavior, have created a rich
opportunity for the integrated measurement of
environmental context and behavior. For
example, combined GPS and accelerometer units
can be used to measure both intensity of
physical activity and the setting in which
this activity occurred.
Through collaboration with other research
groups at the WELL
Center , we developed statistical
machine learning strategies for informing real
time individualized sequences of treatments
(Just-in-Time Adaptive Interventions) and
integrating multimodal data generated from
wearable devices (e.g., fitness trackers,
heart rate monitors). These modeling
strategies have been applied to predict
dietary lapses and affect liability for
patients with eating disorders. See here
for details.
|
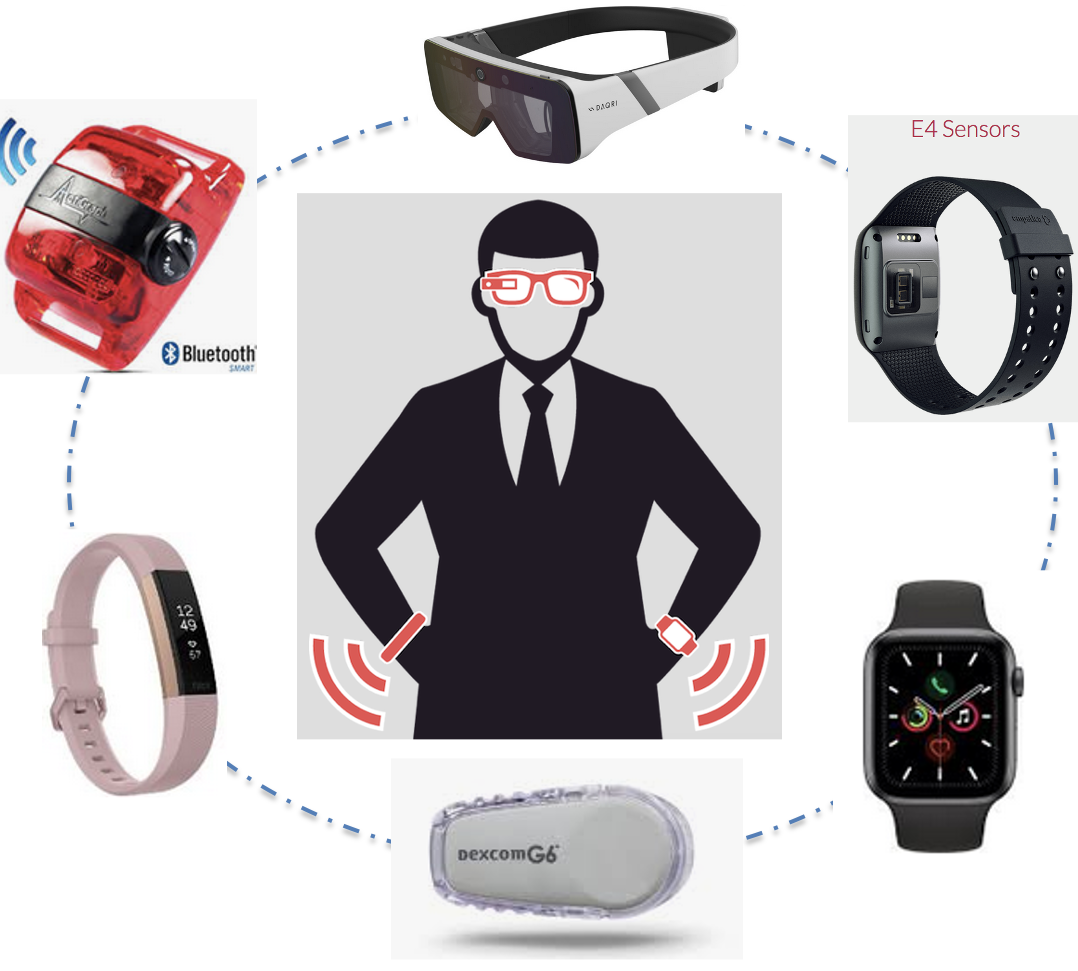
|
Data Mining
As the amount of data being generated is
exploding, we have entered the era of Big
Data. To the extent that data can be analyzed,
we may be able to gain a completely new
perspective on our world, on how people
interact, spend their resources, and organize
their time. Data mining involves using
algorithms and experience to extract patterns
that are either very complex, difficult or
time consuming to identify. In contrast to
traditional hypothesis-driven approaches to
analysis, data mining techniques enables
researchers to assess the predictive value of
all possible combinations of variables in a
data set. Data mining has emerged in recent
years as a major area of statistical research
and practice and is increasingly employed by
psychologists and other behavioral scientists.
In one of our projects, we developed
IMSmining, a free software tool for
automatic selection of potential biomarkers
and discrimination of disease and healthy
groups. This software tool combines functions
of intuitive visualization of imaging mass
spectrometry (IMS) data with advanced data
mining algorithms such as elastic net, sparse
PCA as well as our proposed models (EN4IMS and
WEN). Click here
for more details.
Through collaborations with the WELL
Center, we developed data mining models
for OnTrack,
a therapeutic smartphone app. Data
mining models are built to learn relationships
between triggers and dietary lapses, and
provide real-time prediction when an
individual might be at risk for going off
their diet.
|
High Dimensional
Data
Analysis
In traditional statistical analysis, we assume
many observations and a few, well-chosen
variables. The trend today is towards
more observations but even more so, to much
larger number of variables. If the number of
variables can greatly exceed the number of
observations, we call this type of data
high-dimensional data. High dimensionality
brings noise accumulation, spurious
correlations and incidental homogeneity. The
complexity and high dimensionality of data
pose great challenges and difficulties for
information extraction and data analysis.
Over the past few years, significant
developments have been made in
high-dimensional data analysis, driven
primarily by a broad range of applications in
many fields such as neuroimaging, genomics,
and social network data. In our lab, we have
been focused on areas of feature selection,
classification, regression and dimension
reduction. For example, we developed feature
selection and classification strategies for
brain decoding using fMRI data as well as
imaging mass spectrometry data. See here
for details.
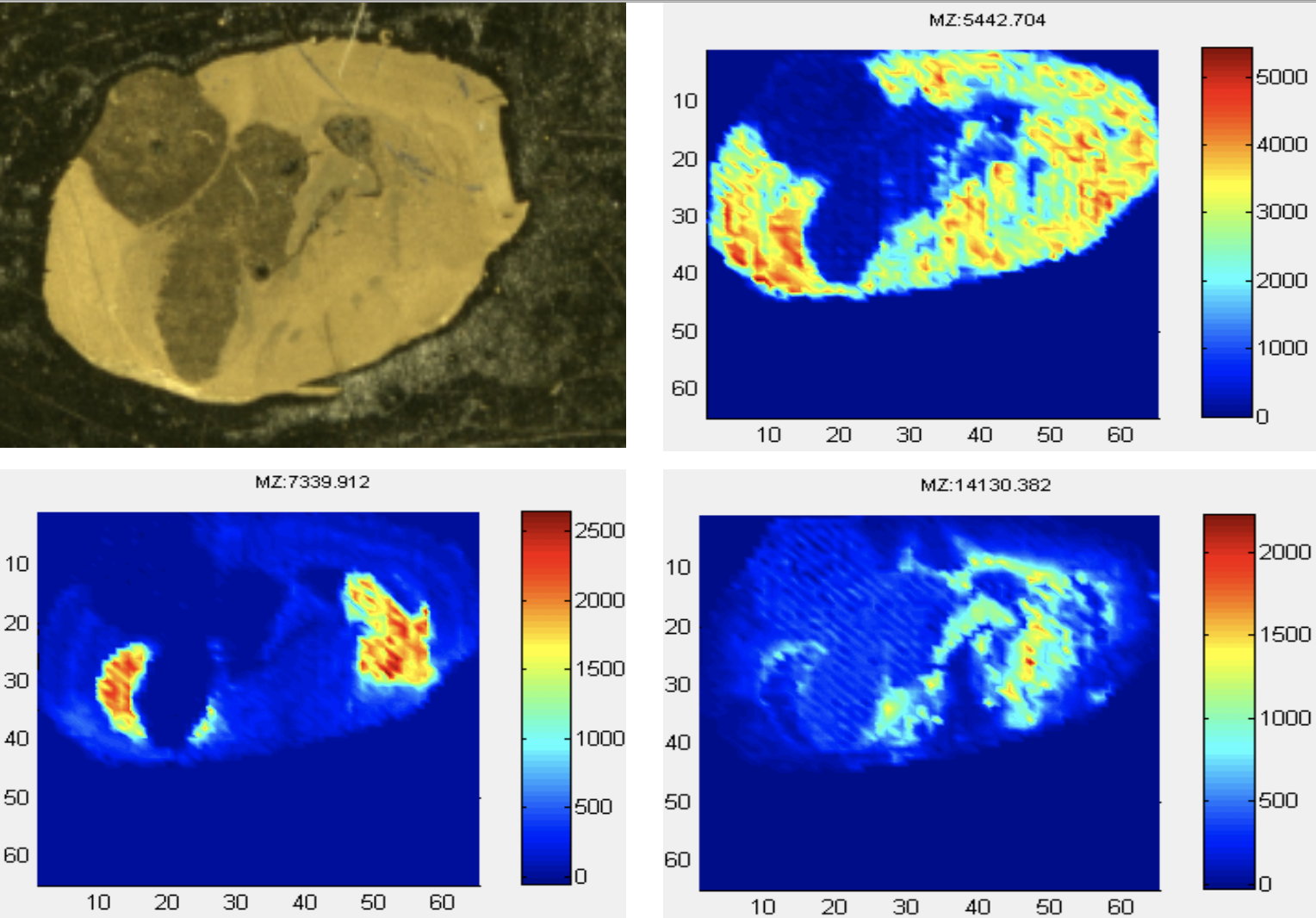
|
Bayesian
Inference
Bayesian inference is a type of statistical
inference in which the probability for a
hypothesis is updated based on Bayes’ theorem as
more information becomes available. A key
element of the Bayesian inference is to treat
parameters as random variables instead of fixed
constants. This approach requires a prior
distribution for each unknown parameters whose
distribution is updated as a posterior
distribution. Bayesian analysis provides a
natural and principal way of combining prior
information with data. In addition, it provides
a flexible setting for a wide range of models
such as hierarchical models.
In one of our projects, we developed a Bayesian
spatially varying coefficient model to
incorporate spatial information among brain
voxels for fMRI data. By incoroporating the
spatial prior information, our approach is shown
to be effective in identifying the truly
localized patterns of the voxels while
maintaining robustness to discover truly
distributed pattern. Click here
for more details.
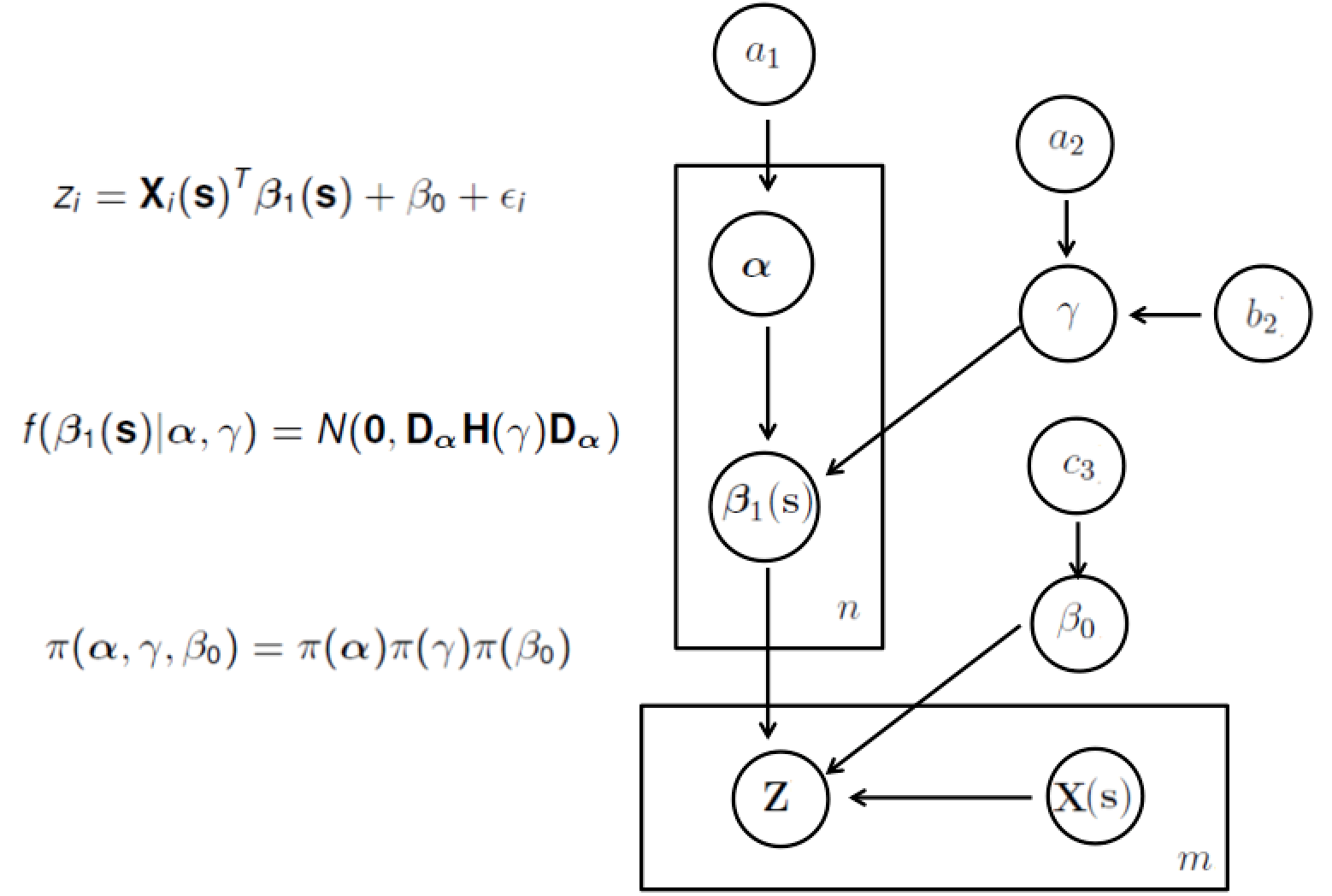
|
Weight Loss
Maintenance
Since I joined the Psychology department at
Drexel, I have been actively involved in several
NIH funded projects on assessment of novel
interventions for weight loss and maintenance. I
collaborate with the WELL
Center and the Lowe
Lab. Some of my working topics include a
test of nutritional interventions to enhance
weight loss maintenance using multilevel
modeling, examination of environmental and
acceptance-based factors for weight loss
maintenance, and a mobile intervention for
dietary lapses using data mining.
|
|